10 Minutes To Pandas
- Pandas in 10 minutes by Wes McKinney www.pydata.orgPyData is an educational program of NumFOCUS, a 501(c)3 non-profit organization in the United States.
- 10 Minutes in pandas 中文翻译. Contribute to Leohc92/10-Minutes-to-pandas development by creating an account on GitHub.
10 Minutes To Pandas Tutorial
10 Minutes to Pandas¶. This is a short introduction to pandas, geared mainly for new users. You can see more complex recipes in the Cookbook. Customarily, we import as follows. The 10 Minutes to pandas is a great place to start learning how to use it for data analysis. Things get a lot more interesting once you’re comfortable with the fundamentals and start with Reshaping and Pivot Tables. 10 Minutes to Pandas (in 5 Minutes) Computer Science, Data Science, Data Structures, Pandas Library, Python, Scripting / By Chris This tutorial provides you a quick and dirty introduction to the most important Pandas features. A popular quickstart to the Pandas library is provided by the official “10 Minutes to Pandas” guide.
The Pandas map( )
function is used to map each value from a Series object to another value using a dictionary/function/Series. It is a convenience function to map values of a Series from one domain to another domain.
Let’s have a look at the documentation of the map
function,
- map is a Series method – operated on top of a Series object.
In the above, pandas.Series.map
takes one major argument, “arg”.
As mentioned in the parameters above, there are 3 different types of possible placeholders for “arg”. In simple they are;
- A Dictionary
- A Function
- An Indexed Series
We’ll explore each of the above argument types in detail. You can use anyone based upon your use-case.
Let’s create a DataFrame that we can use further in the tutorial to explore the map function. The data we have is information about 4 persons;
Each column in the DataFrame is of Series type. So, we can map a dictionary to a column in the DataFrame because the map is a Series method.
From the possible different types of arguments to the map function mentioned above, let’s use the dictionary type in this section. In Machine Learning, the data we provide to create models is always in numerical form. If you observe the “Sex” column’s dtype in the DataFrame below, it’s of String (object) type.
All values of the “Sex” column values are one of the two discrete values – “M” or “F”. “M” representing Male and “F” representing Female. We can’t provide this column to build a Machine Learning model as it’s not of numerical type. So, the use-case is to convert this column to a numerical type. This kind of data is called “Categorical data” in Machine Learning terminology.
We shall use the map function with a dictionary argument to convert the “Sex” column to a numerical data type. This process of converting Categorical data to numerical data is referred to as “Encoding”. As we have only 2 categories this encoding process is called as “Binary Encoding”.
The code for it is,
10 Minutes To Pandas Answer
If you observe the above resultant Series, ‘M’ is mapped to 0 and ‘F’ is mapped to 1 in correspondence to the dictionary.
The above process of mapping using a dictionary can be visualised through the following animated video,
From the possible different types of arguments to the map function mentioned above, let’s use the “Function” type in this section. Let’s achieve the same results of the above dictionary mapping using a Python function.
10 Minutes To Pandas 번역
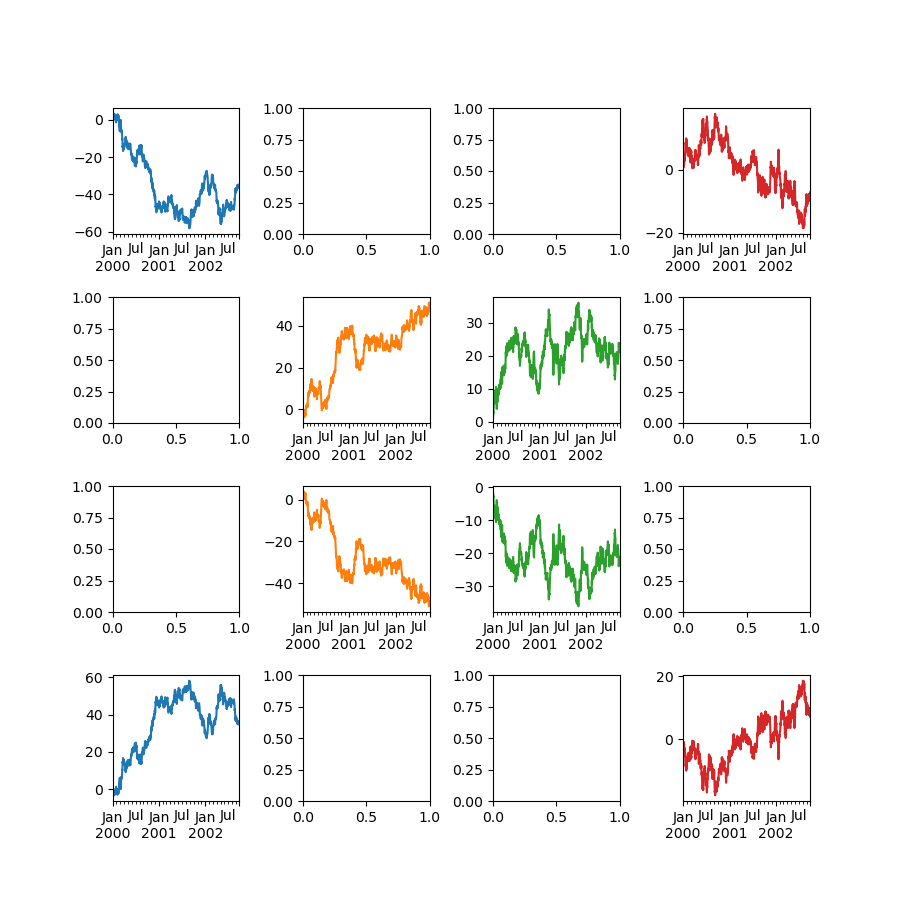
We need to create a function for it at first. The function should take all values in the “Sex” column one by one and convert them to respective integers.
Now let’s use the above function to map it to the “Sex” column.
The code for it is,
The above result is the same as the result of using the dictionary argument. We can check it by comparison;
From the above result, you can see that both results are equal.
The above process of mapping using a function can be visualised through the following animated video,
From the possible different types of arguments to the map function mentioned above, let’s use the “Indexed Series” type in this section. The people in our DataFrame are ready to provide their nicknames to us. Assume that the nicknames are provided in a Series object. We would like to map our “Name” column of the DataFrame to the nicknames. The condition is;
- The index of the nicknames (called) Series should be equal to the “Name” (caller) column values.
Let’s construct the nicknames column below with the above condition,
Let’s map the above created Series to the “Name” column of the Datarame;
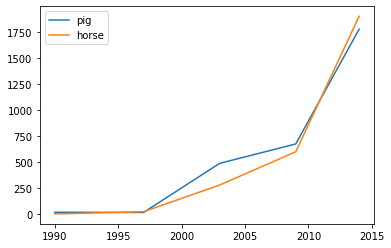
[[watch]] the social dilemma - (2020) full movies & streaming online. The code for it is,
- The major point of observation in applying the map function is – the index of the resultant Series index is equal to the caller index. This is important because we can add the resultant Series to DataFrame as a column.
Let’s add the resultant Series as a “nick_Name” column to the DataFrame,
10 Minutes To Pandas 中文

Panda Commands
The above process of mapping using an indexed Series can be visualised through the following animated video,
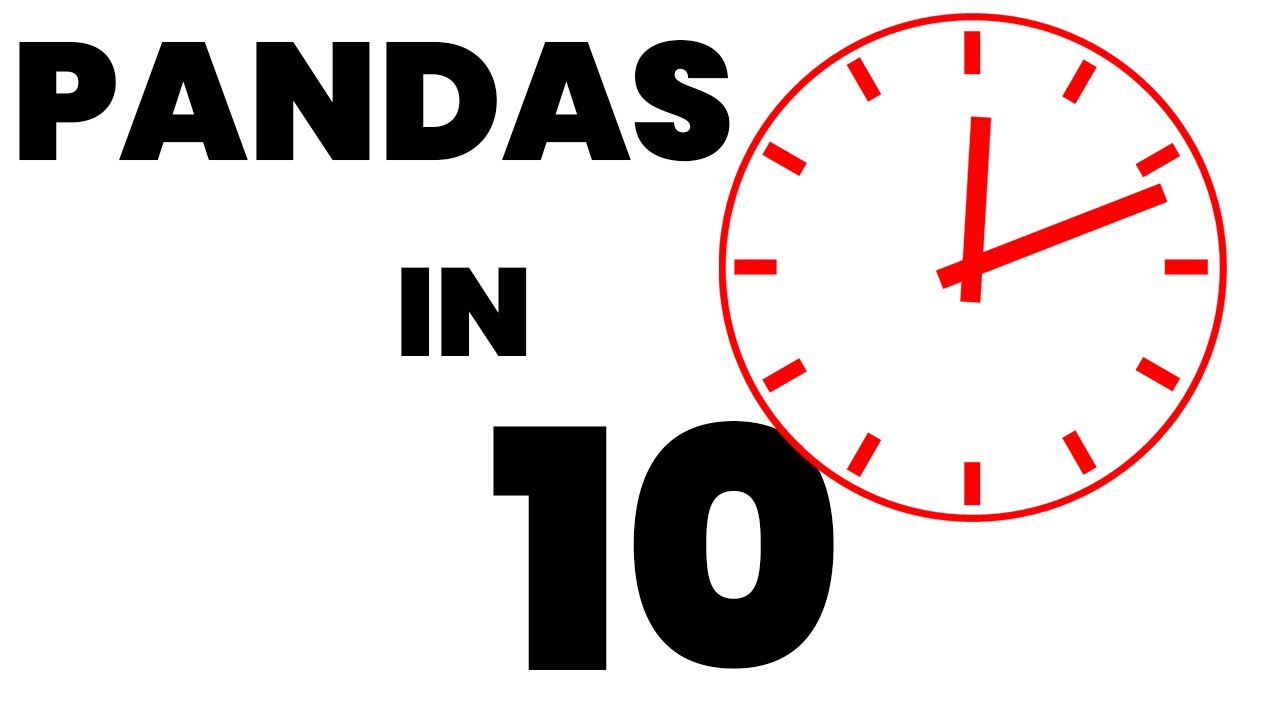
Every single column in a DataFrame is a Series and the map is a Series method. So, we have seen only mapping a single column in the above sections using the Pandas map function. But there are hacks in Pandas to make the map function work for multiple columns. Multiple columns combined together form a DataFrame. There is a process called stacking in Pandas. “Stacking” creates a Series of Series (columns) from a DataFrame. Here, all the columns of DataFrame are stacked as Series to form another Series.
10 Minutes To Pandas Python
We have encoded the “M” and “F” values to 0 and 1 in the previous section. When building Machine Learning models, there are chances where 1 is interpreted as greater than 0 in doing calculations. But, here they are 2 different categories and are not comparable.
So, let’s store the data in a different way in our DataFrame. Let’s dedicate separate columns for male (“M”) and female (“F”). And, we can fill in “Yes” and “No” for a person based upon their gender. This introduces the redundancy of the data but solves our discussed problem above.
It can be done so by the following code,
Now, we shall map the 2 columns “Male” and “Female” to numerical values. To do so, we should take the subset of the DataFrame.
You can observe that we have a DataFrame of two columns above. The main point to note is both of the columns have the same set of possible values.
Thereafter, we will use the stacking hack and map two columns to the numerical values. This can be implemented using the following code,
If you observe the above code and results, the DataFrame is first stacked to form a Series. Then the map method is applied to the stacked Series. FInally unstacking it results in, numerical values replaced DataFrame.
In Machine Learning, there are routines to convert a categorical variable column to multiple discrete numerical columns. Such a process of encoding is termed as One-Hot Encoding in Machine Learning terminology.
We have discussed Pandas apply
function in detail in another tutorial. The map
and apply
functions have some major differences between them. They are;
- The first difference is;
map
is only a Series method.apply
is both the Series and DataFrame method.
- The second difference is;
map
takes dict / Series / function as an argumentapply
takes the only function as an argument
- The third difference is;
map
is an element-wise operation on Seriesapply
is used for complex element-wise operations on Series and DataFrame
- The fourth difference is;
map
is used majorly to map values using a dictionaryapply
is used for applying functions that are not available as vectorized aggregation routines on DataFrames
How To Use Pandas
A map function is used majorly to map values of a Series using a dictionary. Whenever you find any categorical data, you can think of a map method to convert them to numerical values. If you liked this tutorial on the map( )
function and like quiz-based learning, please consider giving it a try to read our Coffee Break Pandas book.
Related Posts
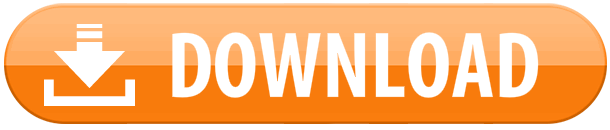